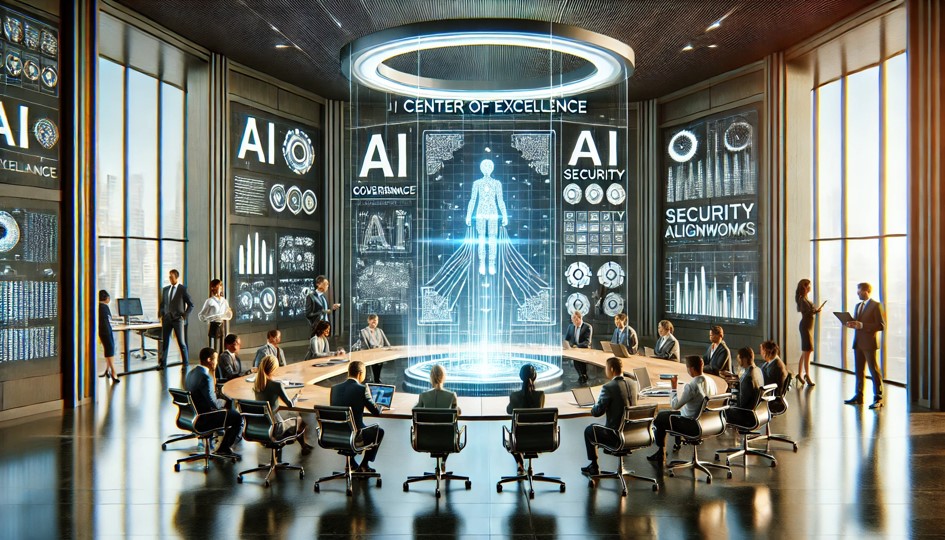
Thoughts on the need for an AI CoE
AI Center of Excellence (AI CoE)
Introduction
This blog argues the case for AI Centers of Excellence (CoEs).
Marc S. Sokol aptly points out that "AI is not just about a ChatGPT-type solution." Discriminative AI, for example, has been around for years, progressing through Gartner's hype cycle—from inflated expectations to disillusionment, and now into the slope of enlightenment with numerous everyday applications. These typically address specific problems (e.g., fingerprint recognition) and are developed by your data scientists and business SMEs. From a risk standpoint, they are well-contained.
Then there’s generative AI (with ChatGPT as just one example), natural language processing (NLP) at scale, Retrieval-Augmented Generation (RAG) architectures, and agentive AI. This new wave of technology is profoundly different. It doesn’t simply open up new use cases; it defies being neatly contained within on-prem solutions or limited to discrete problems managed by in-house analysts. It is increasingly pervasive, rapidly infiltrating all parts of the business process and often relying heavily on third, fourth, and even fifth-party services beyond your direct control or oversight. The potential impact—both positive and negative—on enterprises will be substantial and must be managed thoughtfully. An AI CoE provides a structured way to approach this.
That's my perspective, but what might this mean for you and your organization?
Perhaps the first question to ask yourself is "Why do we need an AI CoE"? To get your head around that, consider:
- Is the adoption of discriminative and generative AI in all aspects of the business both inevitable and non-trivial?
- Are the business benefits—including accelerated innovation, efficiency, improved customer experience (CX), and enhanced brand—strategic?
- Are the efficacy, legal, regulatory, reputation, cyber, and OpEx risks high? Perhaps even existential?
- Are the risks, including the risks associated with not adopting the tech, measured and managed?
- Are the tech and the ecosystems changing at a rate that is difficult for the organization (people and process) to absorb?
- Do I have business operations, customers, employees, or business processes running in the EU? If so, you are required to comply with the EU AI Act when enforcement starts in December of this year. To really answer this, you must have mapped, assessed, and adequately managed all AI processes—even those embedded in third-party systems.
If the answer was 'yes' to most (or all) of these questions, then it makes sense to invest in programs to help the organization manage AI across the enterprise as efficiently and effectively as possible. That's the why.
The what must deliver strategic alignment, rigorous governance, and a culture of continuous measurement, learning, and adaptation.
One way to approach this problem is with an AI CoE. Exactly what that means will vary by organization, but here's a checklist of things that you might consider:
AI Center of Excellence (CoE) Purpose Checklist
In no particular order..
- Strategic Alignment for All AI Initiatives: When change is slow, institutional knowledge can help keep the organization moving toward a common goal. During rapid change, alignment must be explicit, communicated, and reinforced.
- FinOps: A good FP&A person to measure costs, determine what's driving them (users, APIs), and provide forecasting and recommendations for cost control.
- Risk-Based Governance and Compliance: The regulatory landscape is complex and changing fast. Develop, enforce, and audit frameworks to manage risks and ensure compliance with both internal policies and evolving external regulations, including ethics, data privacy, and security.
- Knowledge Dissemination and Training: Cultivating proficiency is crucial. Provide continuous learning and development opportunities to promote AI proficiency across the organization. This is not just for PhDs but encourages critical thinking about data for every team member - An AI driver’s license.
- Security and Privacy by Design: Integrate security from the design phase of AI systems, applying principles like zero trust and MLOpsSec (automated checks for model provenance and poisoning) practices, to safeguard against emerging threats. Automate technical controls for privacy during training, when creating contextual data for RAG embeddings, at prompts, and APIs. Consider scale, multi-modal, and multi-national requirements.
- Innovation and Adaptability: Foster a culture of innovation, exploring new tech and services while remaining adaptable to changing technological and business landscapes. Create safe spaces for innovation that help contain risk.
- Cross-Functional Collaboration: Facilitate collaboration among departments (IT, security, compliance, finance) to ensure AI decisions consider cost, security, compliance, and business needs. This has to be done both inter- and intra-organizationally.
- Stakeholder Engagement: Continuously engage stakeholders to align AI initiatives with business goals through regular communication and collaboration.
- Roadmap for AI Adoption: Create a clear roadmap that aligns AI projects with strategic goals, prioritizing based on potential impact.
Tactics to Consider
Activities (the how) might include:
- Develop a Charter with C-Level Support: Clearly define the AI CoE's purpose, authority, and roles within a highly matrixed team.
- Create a Vision, Strategy, and Pragmatic Plan: Develop a forward-thinking vision, a strategy that outlines behaviors, and a detailed plan with resources, responsibilities, and timelines.
- Engage Stakeholders: Establish continuous communication and collaboration with key stakeholders. Ask "What will make them successful?" and build that into plans. Share success stories.
- Establish Governance and Compliance: Implement and enforce policies to manage AI risks and ensure compliance, with regular audits to maintain standards.
- Provide Training and Resources: Enhance AI proficiency through comprehensive training programs, promoting continuous learning to stay current with AI advancements.
- Embed Security from the Start: Integrate security and privacy into AI systems during the design phase, staying responsive to new threats and updating security measures accordingly.
- Foster a Culture of Innovation: Promote experimentation and exploration of new AI technologies to keep the organization at the forefront of innovation. Define and create sandboxes that enable this to be done safely.
- Identify and Prioritize Business-Driven Use Cases: Resources are limited. Allow playtime in the sandbox but govern spend. Focus large bets on projects with the highest potential for business impact.
- Create a Target Data Architecture: Build a strong data infrastructure to support AI initiatives effectively.
- Manage External Innovation and Relationships: Collaborate with external partners and stay updated on what's happening with tech, products and services, regulatory, and standards changes.
- Develop a Network of AI Champions: Empower key individuals within the organization to advocate for AI adoption.
Conclusion
For most companies, an AI Center of Excellence is not a luxury but a necessity. It will help ensure that investments (time, focus, and money) are strategically aligned, governed, secured, and continuously evolving. It will help the business move faster within the organization's propensity for risk.
One Last Bit of Advice
This is a lot to take in, and it might feel overwhelming. The technology may be new, but the playbook for managing it is not. Maturity within the organization for using and governing AI will be an evolution, not a revolution. This is not a one-off project; it’s a multi-year program. Set reasonable goals and milestones, exceed them, then gradually raise expectations and targets. Rinse and repeat.